When load testing an entire cloud backend, you need to address several concerns that are not necessarily accounted for in component-level testing. Quotas are a good example. Quota issues are difficult to foresee before you actually hit them. And, we needed to consider many quotas! Do we have enough quota to spin up 200 VMs? More specifically, do we have enough for the type of machine (E2, N2, etc.) that we use? And even more specifically, in the cloud region where the project is deployed?
From our partners:
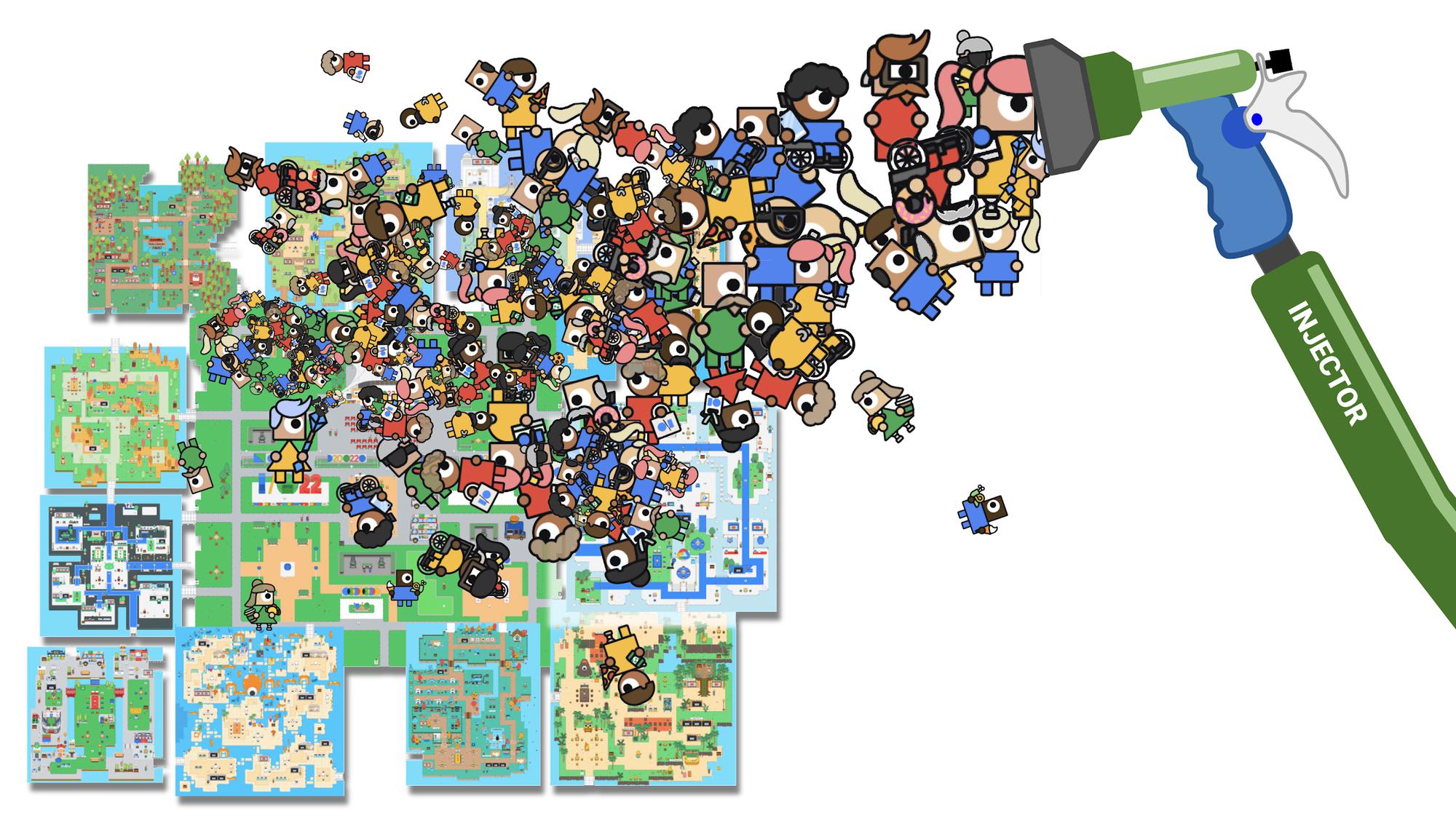
Pouring thousands of bots in the I/O Adventure world
Infrastructure
To design the load test and simulate thousand of attendees, we had to take two key factors into account:
- Attendees communicate from their browser with the cloud backend using WebSockets
- A typical attendee session lasts for at least 15 minutes without disconnecting, which is long-lived compared to some common load testing methodologies more focused on individual HTTP requests
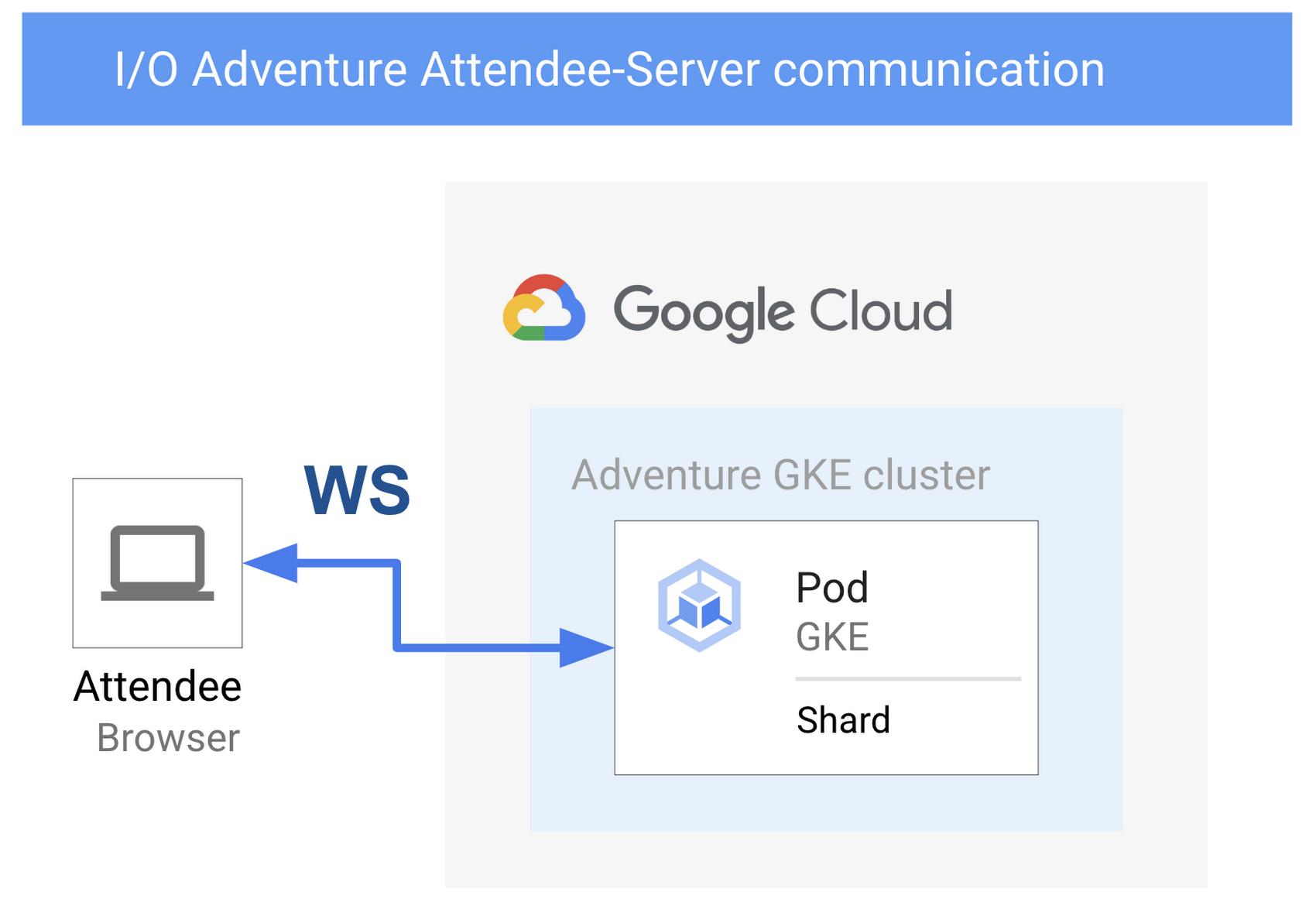
I/O Adventure Attendee-Server communication between attendee’s browser and a GKE server pod, using WebSockets

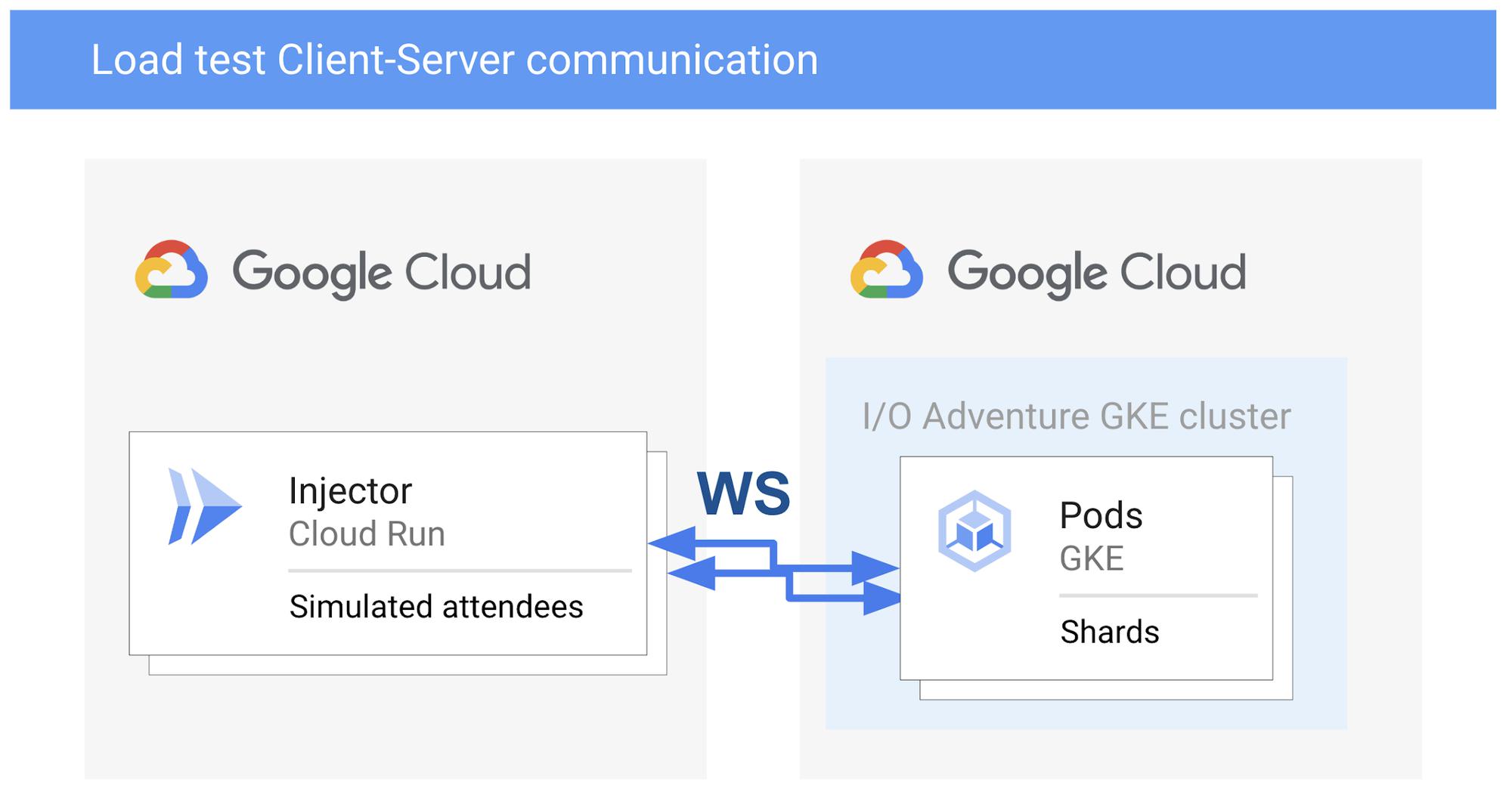
Load test Client-Server communication using Cloud Run communicating via WebSockets with GKE pods
Concurrency
On the backend, the I/O Adventures servers handle thousands of simultaneous attendees by:
- Accepting 500 attendees in each “shard”, where each shard is a server representing a part of the whole conference world, in which attendees can interact with each other;
- Having hundreds of independent, preprovisioned shards;
- Running several shards in each GKE Node;
- Routing each incoming attendee to a shard with free capacity.
On the client side (the load injector), we implemented multiple levels of concurrency:
- Each trigger (e.g. an HTTPS request from my workstation initiated with curl) can launch many concurrent sessions of 15 minutes and wait for their completion.
- Each Cloud Run instance can handle many concurrent triggering requests (maximum concurrent requests per instance).
- Cloud Run automatically starts new instances when the existing instances approach their full capacity. A Cloud Run service can scale to hundreds or thousands of container instances as needed.
- We created an additional Cloud Run service specifically for triggering more simultaneous requests to the main Cloud Run injector as a way to amplify the load test.
Simulating a single attendee story
A simulated “user story” is a load test scenario that consists of logging in, being routed to the GKE pod of a shard, making a few hundred random attendee movements for 15 minutes, and disconnecting.
For this project, I ran the simulation in Cloud Run, and I kicked off the test by issuing a curl command from my laptop. In this setup, the scenario (story) initiates a connection as a WebSocket client, and the pods are WebSocket servers.
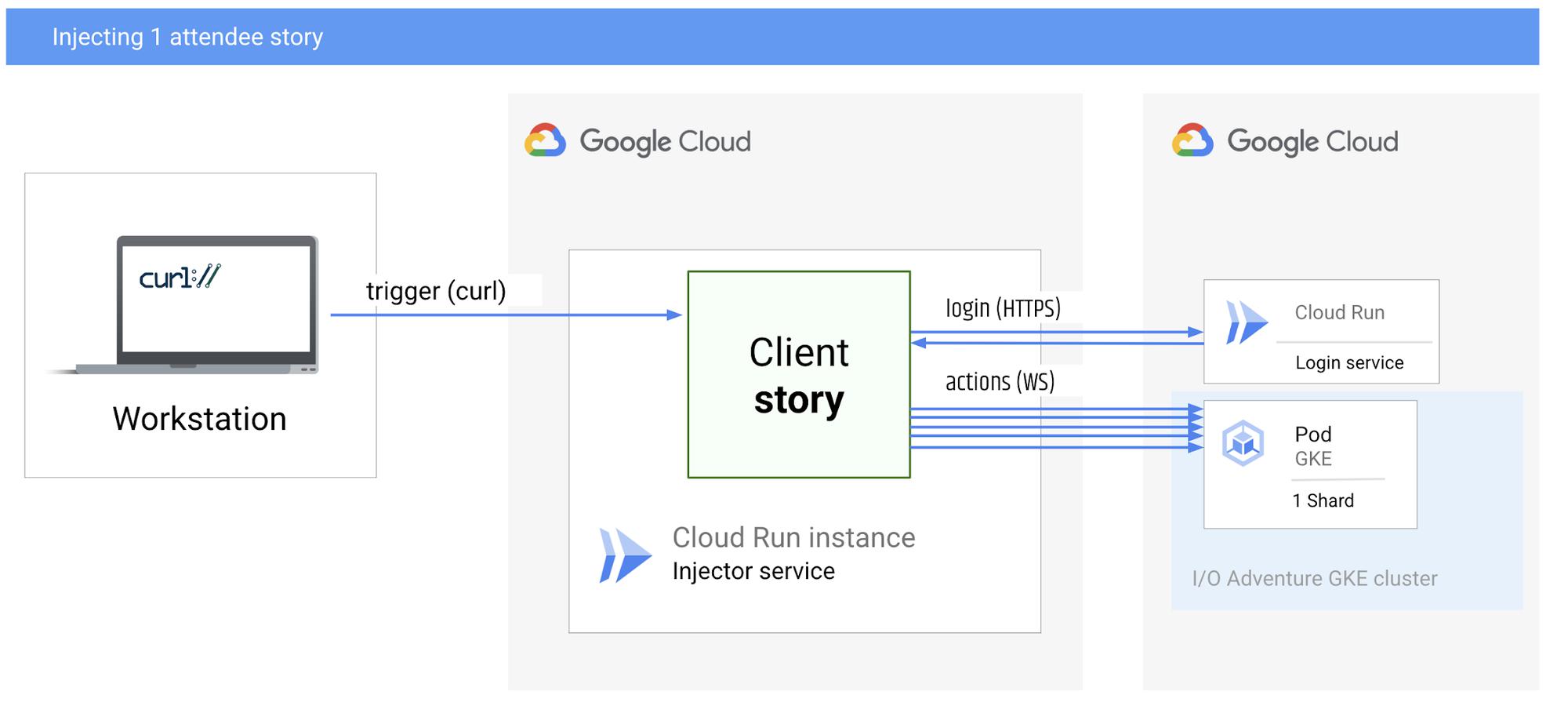
Injecting one attendee stroy
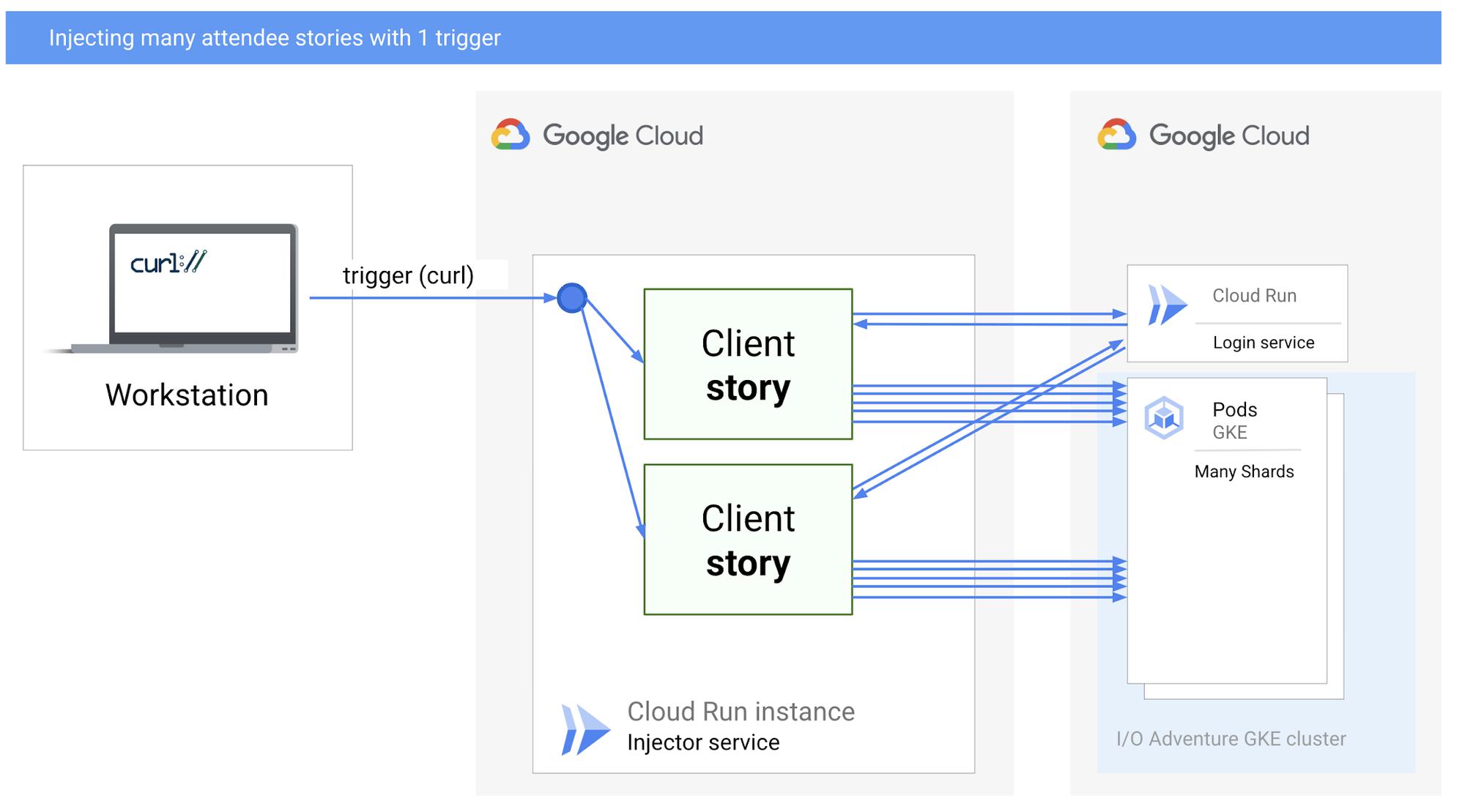
Injecting many attendee stories with one trigger
for i in {1..10}; do
curl -X POST "https://fancy-load-test.run.app" &
done
wait
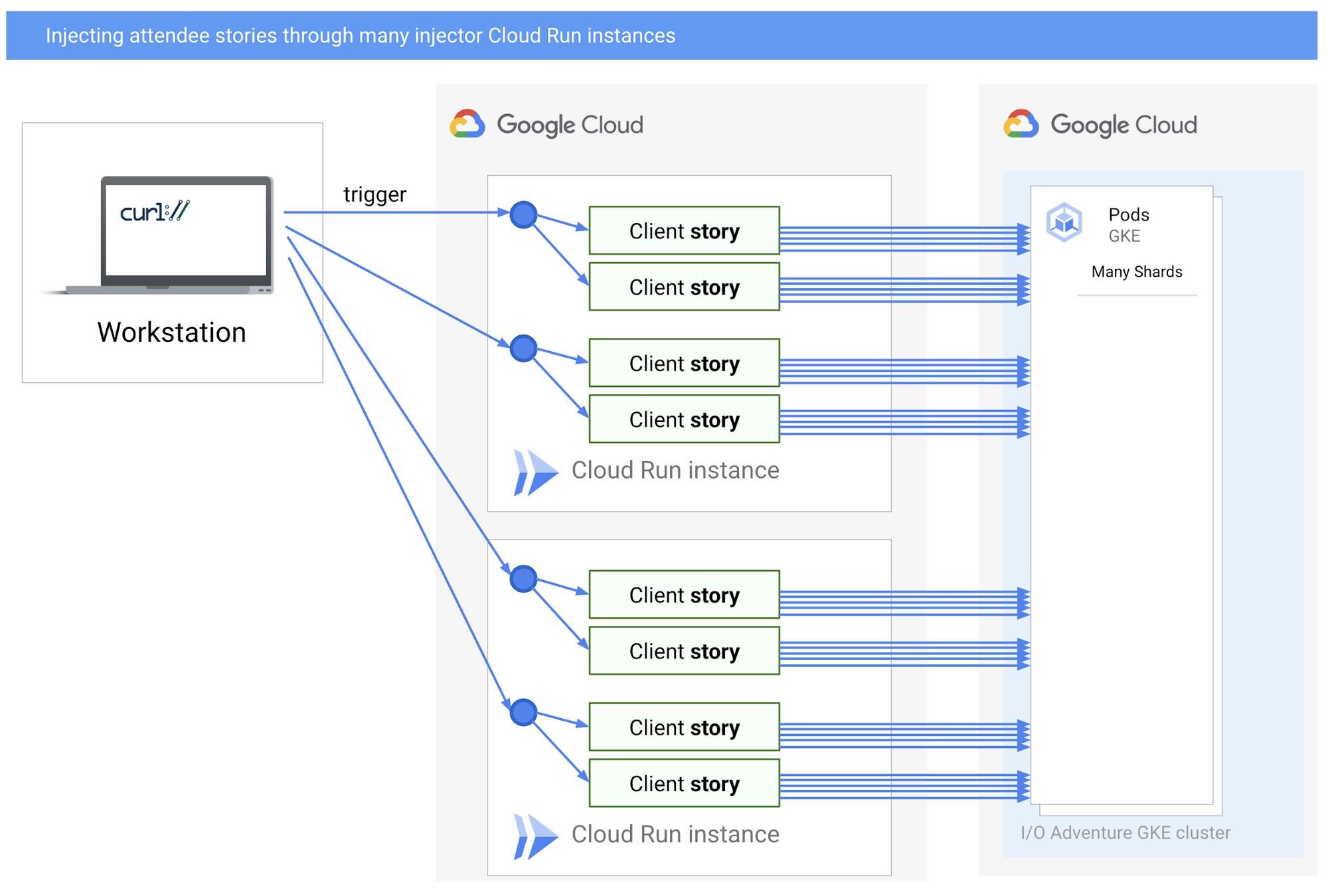
Injecting attendee stories through many curl requests, triggering many Cloud Run injector instances(Login service details omitted for clarity.)
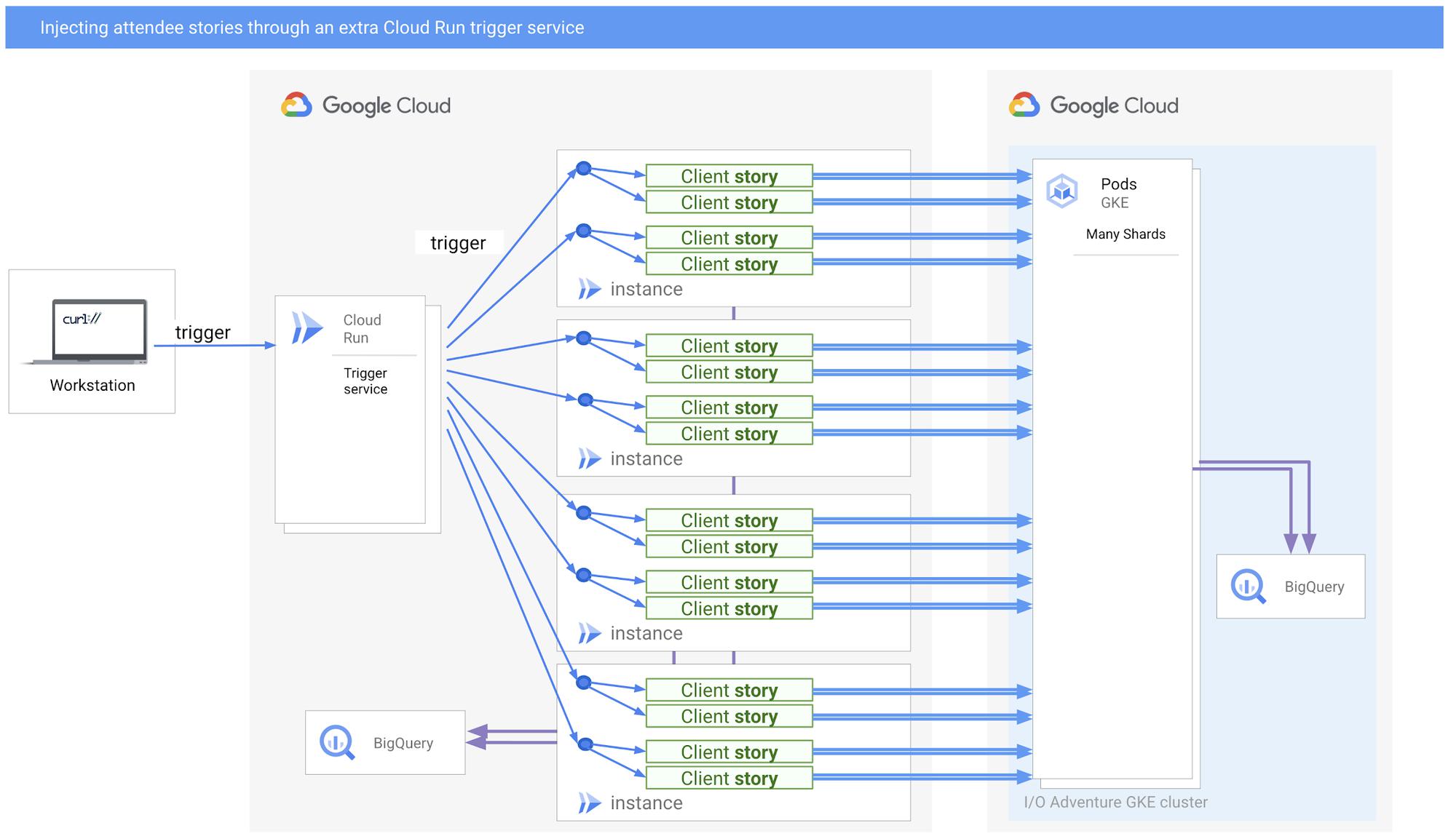
Injecting attendee stories through an extra Cloud Run trigger service
Measuring the success rate
Unlike HTTP requests, which have an explicit response code, WebSocket messages are unidirectional and by default don’t expect an acknowledgement.
To keep track of how many stories have run successfully to completion, we wrote a few events (login, start, finish…) to the standard output and activated a logging sink to stream all of the logs to BigQuery. The events were logged from the point of view of the clients (the injector) and from the point of view of the servers (GKE pods).
This made it very convenient, with aggregate SQL queries, to:
- make sure that at least 99% of all the stories did finish successfully, and
- make sure the stories did not take more time than expected.
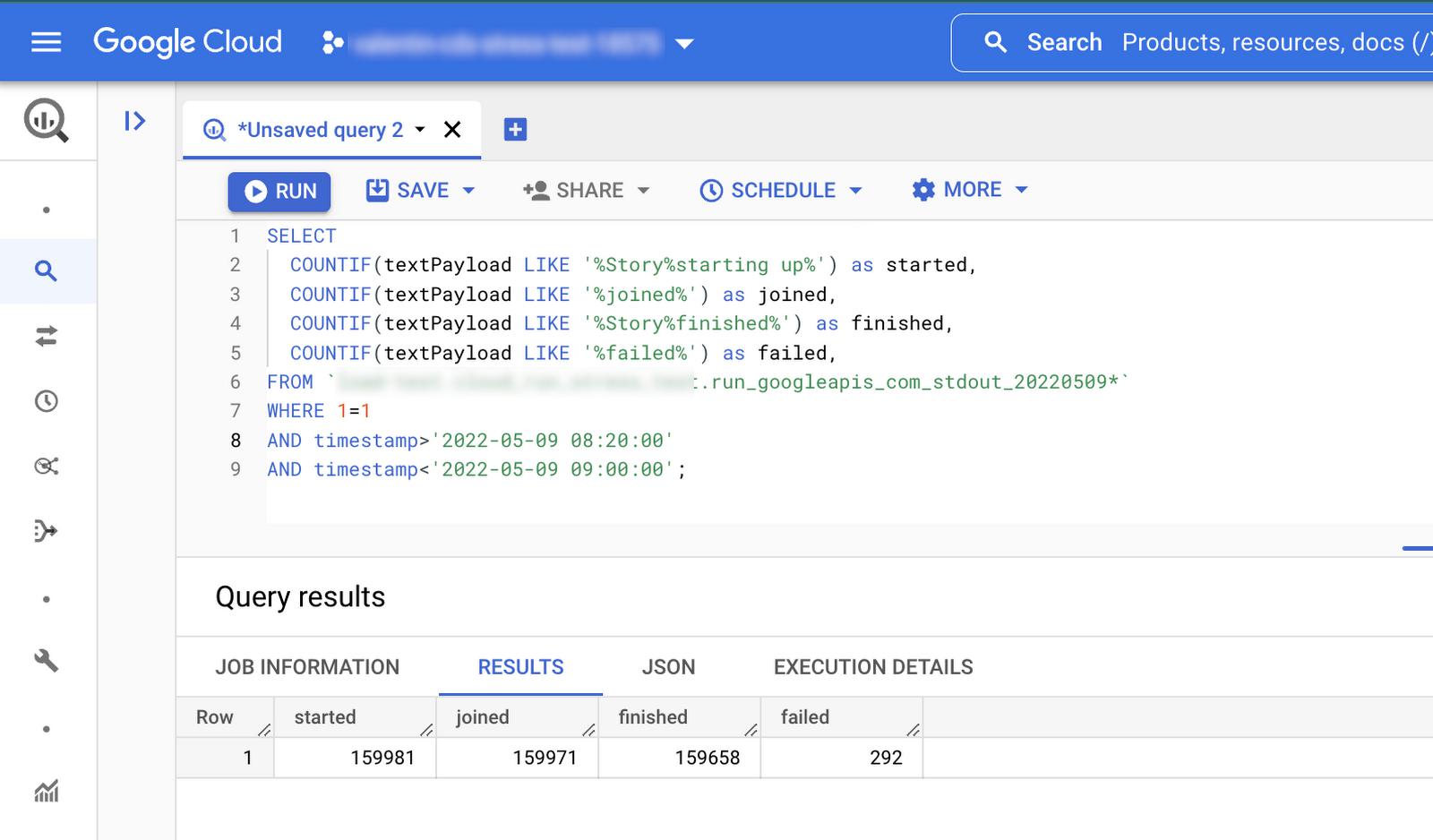
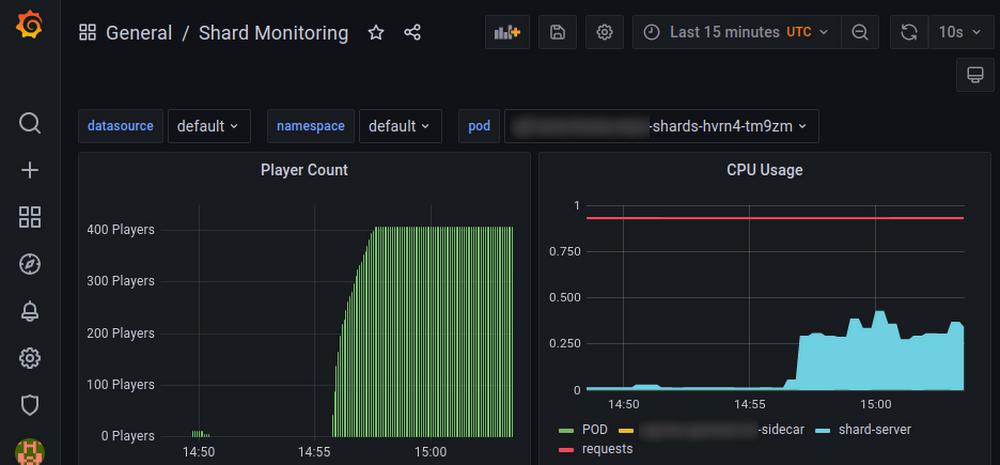
Visual check
As a bonus, it was very fun to connect as a “real” attendee and watch hundreds of bots running everywhere!
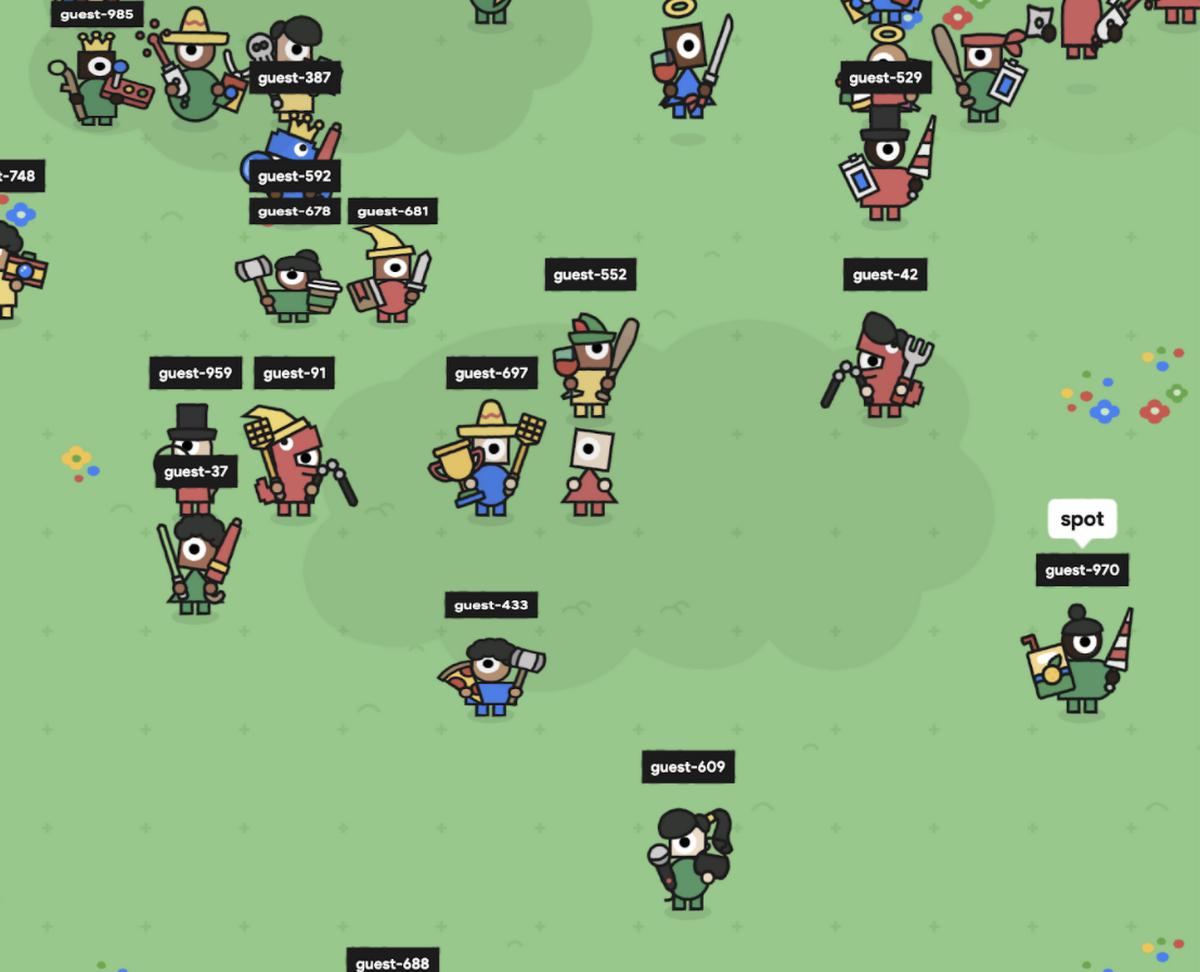
Results
With a total of 4000 triggers for 40 stories each, and a max concurrency of 40 requests per Cloud Run instance, our tests used just over 100 instances and successfully injected 160,000 simultaneous active attendees. We ran this load test script several times over a few days, for a total cost of about $100. The test took advantage of the full capacity of all of the server CPU cores (used by the GKE cluster) that our quota allowed. Mission accomplished!
We learned that:
- The cost of the load test was acceptable.
- The quotas we needed to raise were the number of specific CPU cores and the number of external IPv4 addresses.
- Our platform would successfully sustain a load target of 160K attendees.
During the actual event, the peak traffic turned out to be less than the maximum supported load. (As a result, no attendees had to wait in the queue that we had implemented.) Following our tests, we were confident that the backend would handle the target load without any major issues, and it did.
Of course, Cloud Run and Cloud Run Jobs can handle many types of workloads, not only website backends and load tests. Take some time to explore them further and think about where you can put them to use in your own workflows!
By: Valentin Deleplace (Google Cloud Developer Advocate)
Source: Google Cloud Blog
For enquiries, product placements, sponsorships, and collaborations, connect with us at [email protected]. We'd love to hear from you!
Our humans need coffee too! Your support is highly appreciated, thank you!